Transparency is the new normal for HR

Every few years, the business zeitgeist reframes our conversation around a key concept or technology. Today is the era of Artificial Intelligence. Although somewhat cliched, AI has been named, the new oil, gold, and oxygen. The rate of innovation in AI and how data is processed and analyzed has surpassed any other innovation since the invention of the internet.
I think that sometimes the noise about advances in technology drowns out more important conversations: what are we using technology to achieve? Because AI is just a tool or a means to an end. Transparency is the end goal and AI can help get us there.
The Case for Transparency
Let's start with a definition of transparency and how it relates to HR. The strict definition of transparency refers to the ability to see through something such as glass. In the extreme, transparency without filters would mean that no information is private or confidential, which is not a desirable state. For the purposes of this article, I will use a more nuanced definition: visibility into the behavior of employees as it relates to their well-being and performance and access to data for informed decision-making.
According to Forrester, by 2030 almost three-quarters of the workforce will be made up of Millennial and Generation Z workers. Raised on a diet of social media and instant communications and gratification. The “sharing generation” expects to understand how decisions are made and want to understand the criteria used by managers for decisions relating to compensation and promotion We are witnessing a U-turn in attitudes as older workers were accustomed to hierarchical organizational structures where decisions are made at a higher level and discussions about compensation are considered private.
The shift to transparency is reflected in how Glassdoor has become a major force in the labor market. Each month, millions of visitors access reviews of a company’s culture, interview processes, and compensation levels. Sourced anonymously, employers such as Yelp, Papa John’s, and Amazon have been forced to make leadership changes and policy or compensation reforms based on Glassdoor activities.
In parallel, there are other demands for change coming from multiple directions as more shareholders, investment firms, and asset managers recognize that a strong and diverse culture is good for business and conversely, inequalities and toxic cultures can reduce long-term value. According to Anne E. Robinson of investor Vanguard, “We believe that diversity, equity, and inclusion risks are equally as disruptive as climate-related risks.”
Whereas the analysts evaluating the merger of Exxon and Mobil in 1972 were not looking for systemic labor inequalities, today there are specific methodologies to evaluate DEI as part of Mergers and Acquisitions due diligence processes.
Regulatory authorities are becoming more proactive. In 2020, the SEC instituted a Human Capital Disclosure Requirement in Form 10K for public companies. Metrics include diversity and inclusion, employee engagement, and voluntary turnover. Several US states have started mandating pay transparency reporting for employees who work in their states including California and New York.
Employee surveys as a flawed analytic resource
If transparency is the goal, are there sufficient tools to make it a reality? For many organizations, the go-to HR analytics tool is the employee survey. More often than not, employee survey data is used as measurements of engagement, morale, and job satisfaction.
Unfortunately, there are widely recognized limitations. At best employee surveys show trends that do little more than scratch the surface of what’s happening in the organization. At worst, response bias and design limitations undermine the accuracy or efficacy of the research.
The result? Surveys provide some interesting information but cannot be relied upon for transparency.
Is HRIS a goldmine or Pandora’s box?
An alternative to surveys is the analysis of the Human Resources Information System or HRIS data. HRIS data typically includes an employee’s personal data, performance ratings, compensation, and training records. Yet for some, HRIS is viewed as Pandora’s box, and there is a reluctance to dig deep and find the answer to difficult questions.
There are legitimate concerns about how to access and use this data. The HRIS contains extremely sensitive records of pay inequalities by different population groups, employee complaints, and reasons for termination. The misuse of this information could be harmful to the organization and its reputation.
There is a long list of well-known reasons to tread carefully: privacy, ethics, accuracy, compliance, etc. But is it possible that the guardrails around HRIS are so high that we miss out on the opportunity to resolve difficult situations and re-rebalance bias and inequality? Transparency without data is like a car without fuel.
AI as an Enabler of Transparency
For context, let’s look at why AI is so critical today. The rise of cloud computing platforms and the availability of scalable and cost-effective infrastructure have made it easier for organizations to store, process, and analyze massive amounts of data required for AI applications.
No longer AI confined to academia, AI has become a staple component of product development, contact center automation, and service operations optimization across multiple industries.
We are already seeing AI used for analytics, although not necessarily within the HR function. According to McKinsey research, AI plays a significant role in risk modeling and fraud analytics, and sales and demand forecasting. Today AI is used by Finance for currency hedging and financial projections and by Sales for revenue estimates.
For many in HR, AI is outside the comfort zone
So how do AI and Transparency fit into the HR domain? Transparency is not just about the current state of the organization or visualization of HRIS data. The ultimate value lies in the predictive nature of AI. The next frontier of transparency is the visibility into talent vulnerabilities including attrition risk or burnout at both team and individual levels.
Yet despite the opportunity to use AI, McKinsey research shows that HR is lagging in its adoption. HR seems to be holding back for (at least) two crucial reasons.
First, as with HRIS data, there are well-known concerns about employee privacy. Data that is misused or ends up in the wrong hands could have negative consequences and that’s a bridge too far when HR does not have in-house domain subject expertise. Second, HR involves managing complex human processes, which are subjective and require a nuanced understanding of human nature. Applying a Machine Learning algorithm to people data goes well beyond the comfort zone far for many HR executives.
But change is happening quickly. With more organizations embarking on their Transparency journeys (albeit at different paces), we are seeing HR in catch-up mode.
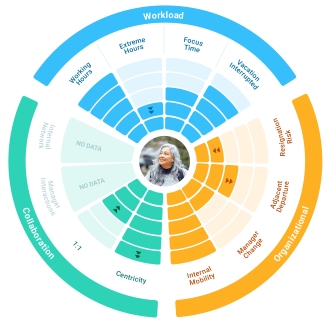
The Journey to HR Transparency
Adopting HR transparency starts with a shift in mindset and reexamining some of these common assumptions. Yes, there are challenges and risks with AI and data, but this is not unique to HR.
The CFO who is responsible for cash flow forecasts that have a material effect on a company’s share price is likely using AI based tools on sensitive financial data. Data encryption, controlled access, and secure storage are standard practices today, whether the source of the underlying data is employee compensation, customer credit card, or sales pipeline. In this regard, HR is no different from other parts of the organization.
I would also like to challenge the narrative that every employee is different and therefore, there is no way to apply an “arbitrary” algorithm to understand their burnout, wellness, or flight risk.
To explore this topic, let’s review the data science of how AI is used to analyze HR data at a high level. Employees generate digital footprints with the use of email, Slack, calendar meetings and any other IT system that we use at work. The footprint is the data that is stored in IT systems. Algorithms are applied to this data and are trained to first learn the expected patterns of behavior for the organization. The algorithm can then classify expected outcomes or identify variances from the normalized behavior. In this way, we can compare one team's behavior relative to other teams or the overall company, or current behavior relative to historic behaviors and performance. AI can compare one team with another or to the overall company. To summarize, once AI recognizes a pattern, it can tell if it’s broken.
An AI algorithm can train on dozens or even hundreds of variables, including HRIS and survey data. In this way, communication and collaboration patterns for specific employee populations can be analyzed. The graph below is illustrative of the output from an algorithm that has learned workload patterns for an entire company.
When a team experiences a spike in extreme hours worked, the algorithm will recognize whether the team has returned to a typical work pattern or whether there are signs of overwork. With this level of transparency, managers can adjust workloads or request more resources if the team workload becomes unsustainable.
Deep HR transparency comes when AI can learn from multiple data sources. In the case of extreme hours worked, analysis of communication patterns alone may not be sufficient to accurately predict burnout. That’s because burnout is also a function of employee age, length of employment, and level of seniority, and could potentially be well over 100 other variables that the human mind would not even think of. The power of AI is that it can simultaneously evaluate vast amounts of data variables for a large population group and provide analysis of complex organizations. Although we often rely on gut feeling, AI is often a more accurate predictor of behavior.

AI is a science, but science isn’t perfect
Returning to the concern that when it comes to human behavior, there are too many complex variables and unknowns to feed an equation. For example, an employee’s assessed resignation risk was extremely low, yet they resign because of a family relocation.
The simple answer here is to acknowledge that AI is not always perfect, and it is used to identify the likelihood of an event, but always, with a certain level of probability. For instance, in the case of using AI for fraud detection, algorithms are trained to learn the credit card shopping patterns of individuals and groups. If a cardholder from Michigan makes a large purchase in Tokyo, that transaction may be flagged as potential fraud. Perhaps in this individual’s case, the transaction was legitimate, but the algorithm will recognize the size and location of transactions as abnormal and might classify it as fraudulent.
Similarly, AI can flag an emerging HR risk, but that does not mean it will certainly occur.
Conclusion
Technology is changing at a rapid pace alongside our appetite for data-driven decision-making. Concepts that were the subject of sci-fi just a few years ago are now mainstream. HR Transparency is not a simple topic. There are legal, policy, and data security issues that need to be considered carefully.
But in my opinion, the end goal is worthwhile: giving employees a greater understanding of how organizational decisions are made and providing the organization with more information about what's happening (and what could happen without corrective behavior) with employees.
Related posts
More insights from Watercooler's team of experts.