HR Analytics: Go Big or Go Home

Sully is a critically acclaimed movie that tells the story of Chesley "Sully" Sullenberger. Tom Hanks plays the pilot of US Airlines Flight 1549 who, based on gut feeling, ignores flight traffic control and lands the plane on the Hudson River. The public investigation into this decision dramatizes a conflict between his intuition and a computer simulation. Sully is exonerated, and human intuition wins out.
What makes the movie appealing today? Machine Learning and Artificial Intelligence play an important part of our decision-making. Whether we like it or not. Sully entertains by tapping into our nostalgia for simpler times.
This article addresses the intersection of human intuition and data-driven People Analytics. Without a neatly-wrapped Hollywood ending, it’s a messy topic with no simple answers.
I will break with convention and skip to the ending. The adoption of data-driven analytics in Human Capital Management is unstoppable.
Post Covid and in a difficult economic environment, we are at an inflection point. The choice is go big or give up and go home.
A Tale of Two Employees
Let’s start with a couple of employee categorizations derived from data-driven People Analytics. Meet two (fictitious) colleagues – Jessie and Rene.
#1 Isolated Employee
Jessie is becoming disengaged. Over time, she is sending and receiving fewer Slack messages than her team members. There is also a spike in the number of 1:1 meetings canceled by her manager.
Jessie is considered a High Isolation Risk. This is due to the combination of declining communications and a more distant relationship with her manager. A deterioration in her well-being is not inevitable, and without an intervention, it is increasingly likely.
#2 Potential Leader
Rene is considered a go-to person in her group, readily available to answer questions, help a colleague or coordinate with other teams. She has extended her network beyond the organization by forming relationships with key customers.
If Rene were to leave, it could harm the team’s morale and productivity. She should be recognized and nurtured as a future manager.
How it Works: The Endless Possibilities of Machine Learning
Terabytes of data are extracted from HRIS, IT and Communication Systems.
Machine Learning algorithms analyze 100’s (or 1,000’s) of data variables. Variables include meetings scheduled, emails sent/received, Slack messages, vacation days utilized, organizational changes (for example, appointment of a new manager) or adjacent departures.
The algorithms recognize patterns of data behavior for a particular population (company, team, local office, etc.). In our example, the algorithm compares the digital behavior of Jessie and Rene because they are part of the same team.
The next step is to determine the relevance and weighting of each variable at an individual level.
More clarity or context is gained when an event or alert (e.g., Isolation Risk is identified) triggers a micro-survey.
The visualizations below capture just one data variable - Slack Messages - for Jessie.
It's not only important to detect a decline in communication frequency. The algorithm also compares Jessie’s pattern with those of her team. This reveals that she is an outlier in regard to inclusion. This data correlates with a decline in the number of emails where she is cc'd by colleagues, a further sign of isolation.
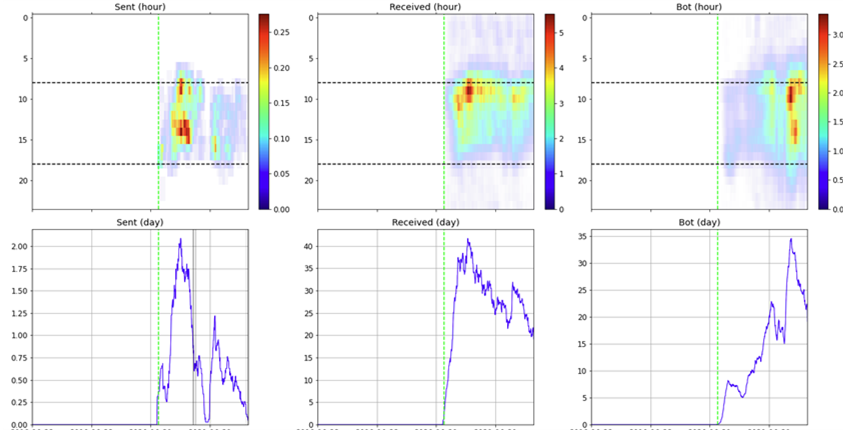
Conversely, Rene stands out because of the strength of her relationships compared to what is expected for her level of seniority. The algorithm first learns the behavior of all employees. It then assigns a typical network strength for each seniority level. Rene acts like leaders with higher seniority designations in the HRIS.
These are just two examples. Other signals include problematic onboarding processes, resignation predictions of key employees, and burnout for underrepresented populations.
If the goal is to use data as a tool to safeguard employee well-being, the possibilities and applications are endless.
…..Made Possible by Innovations in Data Science
Covid lockdowns forced us to change how we collaborate and communicate. The move to Zoom, Slack etc., has generated employee-level digital footprints. These are the primary signals of engagement and well-being.
In the past, it was not feasible to decipher these signals. Teams of analysts had to sift through massive quantities of data to find them. Now, due to advances in data science, a granular level of employee listening is accessible to HR.
Automated Machine Learning or AutoML is a breakthrough in data science. Automation is applied to repetitive and labor-intensive tasks previously performed by data scientists. Even with scarce people resources and tight budgets, fewer specialized data scientists are now needed if the right tools are used.
Gartner refers to Citizen Data Scientist as “a person who creates or generates models that leverage predictive or prescriptive analytics, but whose primary job function is outside of the field of statistics and analytics." Innovations in data science allow us to go the last mile and put analytics insights into the hands of end-users.
People Analytics is similar to other business areas heavily dependent on data science. There is a trend within multiple industries to develop analytics tools for the Citizen Data Scientist. A factory manager today gets equipment safety alerts triggered by Machine Learning algorithms.
Perhaps the HR Citizen Data Scientist role is not that far off.
The Elephants in the Room: Ethics and Privacy
The proverbial elephant in the room. Any discussions about Data and HR lead to the inevitable concerns about employee privacy and data security.
Let’s address the topic head-on.
I propose that we rephrase the conversation. It’s not about whether or not to use HR (or other) data, What's most important is that data is not used in the wrong way.
Every organization has governance for the access and use of information. This long list includes Intellectual Property, customer credit card or even IT system passwords. In each case, there are carefully built solutions to ensure the protection of these assets.
As with other information, there should already be protocols in place to protect employee privacy and control access to HRIS data. Just as we limit access to payroll data, similar restrictions can (and should) be applied to People Analytics. In short, the privacy and security problems are real, and solutions may already exist.
The larger elephant in the room is the fear of unintentional consequences. A Pandora's Box could be stuffed with more analysis than we can handle. Burnout, isolation, toxic work environments, failed onboarding, etc.
This is information that requires us to act.
Identifying the problem is the (relatively) easy part but there is no formulaic fix to human behavior.
Of primary concern are the guardrails needed to protect employees. Let’s use a scenario where a People Analytics tool identifies a toxic team environment using a Machine Learning algorithm.
The ethical challenge is twofold.
First, there is a risk of retribution or misuse of power. We need employee protection from leaders seeking to penalize (perceived) negative employee behavior.
Second, care is needed that this manager not be singled out and penalized solely based on an algorithm-generated analysis.
This requires consideration and sensitivity towards people managers themselves. What are the expectations from managers who are also under pressure due to their own deliverables, goals, and commitments? For instance, the front-line manager responsible for a team's well-being may also be identified as a candidate for high burnout risk. We all know managers who simply lack the experience to help an employee navigate through challenging periods.
This is where the hard work begins.
The original article appeared in TroopHR: https://www.troophr.com/blog/data-driven-people-analytics-go-big-or-go-home
Related posts
More insights from Watercooler's team of experts.