Half of LGBTQ+ workers face discrimination.

June is Pride Month. Follow some corporate posts on LinkedIn and it may seem that thanks to progress, we have evolved to the point of celebrating our differences. Work is a tolerant place, a level playing field open to all.
Speak to a person who identifies as LGBTQ(particularly transgendered), and a very different narrative emerges. In a 2022 study of US employees, half of all LGBTQI+ adults surveyed experienced some form of discrimination in the last year.
Today there is a lot of hype about AI. This article explores the role (and limitations) of using AI in addressing the issue of LGBTQ+ discrimination.
The gap between law and reality
In 2020, the US Supreme Court ruled that employment discrimination against LGBTQ workers is prohibited by the Title VII of the Civil Rights Act.
As defined by Sexual Orientation and Gender Identity (SOGI) Discrimination, the law forbids discrimination “when it comes to any aspect of employment, including hiring, firing, pay, job assignments, promotions, layoff, training, fringe benefits, and any other term or condition of employment.”
Unfortunately, most of us live in a world where these laws are not always followed as they should be. There is a wide gap between legal protections and what’s happening in the workplace, which explains why only a third of LGBTQ+ employees (below the level of senior manager) reported being out with most of their colleagues.
We also see evidence of LGBTQ+ discrimination in all levels of management. A study by McKinsey shows that while 5.1% of US women and 3.9% of US men identify as LGBTQ+, their representation in corporate America is significantly lower. It is particularly concerning to note the low representation of LGBTQ+ women in senior levels of management.
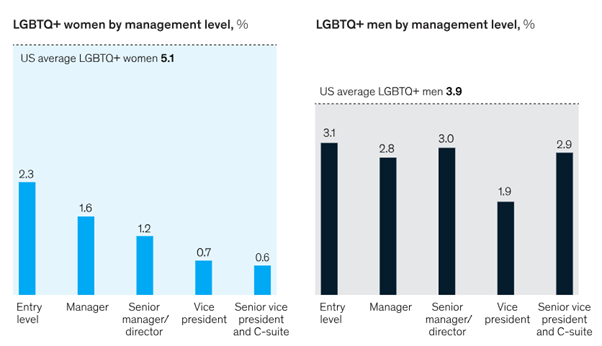
Source: McKinsey& Company
Putting aside decades of progress, even with legal and policy protections in place, we need to admit that bias and discrimination are significant problems. Pretending otherwise does a disservice to those most at risk.
The Role of AI in combatting discrimination
It wasn’t that long ago that Artificial Intelligence was either relegated to academia or sci-fi entertainment. Today, it seems that we have gone in the other direction and that AI is pervasive.
As a practitioner of AI, it sometimes feels that people expect AI to do for humanity what humanity has been unable to do for itself. Solve global warming, rid the world of disease, and change our behavior.
Before identifying areas where AI can play a role in addressing discrimination, let me start off by stating the obvious: AI is a tool. If used correctly, it can impact our lives in positive and meaningful ways. But AI is not going to make us different people; it won’t force people to be more tolerant or remove bias in the workforce.
At the same time, AI can play a significant role today: AI can be used to uncover patterns of discriminatory behavior hidden deep within an organization.
It starts with data, masses of data. Terabytes, Petabytes, and Exabytes are generated by various IT systems we all use on a day-to-day basis. In most cases, this data is captured and stored by IT departments but not used for any functional purpose.
With apologies to my data analytics colleagues for oversimplifying the science, Machine Learning algorithms can be trained to analyze large datasets and extract tiny conclusions (like examining the ocean to find a rogue wave).
Here are some specific scenarios where AI can be used to address LGBTQ+ discrimination:
1. Denying promotions or not receiving pay increases
Machine Learning can be applied to historic data by a company with 200 employees.
The Machine Learning modeling process is performed without informing the algorithm (or labeling data) as to which employees are identified as LGBTQ+. The algorithm will analyze multiple data points from the HRIS including tenure, time since the last promotion, and performance review, and can then make predictions as to which employees are the best candidate for promotion or pay increases. The list generated by the Machine Learning algorithm can be compared to the actual list of promotions and pay increases. If the actual promotions do not match what the algorithm predicted – and if LGBTQ+ employees who were predicted to be promoted were not, this is a red flag.
Does it mean that the reason that people were not promoted despite the Machine Learning prediction was because of discrimination? Not necessarily. There are numerous valid reasons why someone was not promoted even though a model suggested otherwise or did not receive the pay increase predicted.
At the same time, this type of data can be used as the basis to explore whether biases are occurring, as long as the analysis is done carefully and without assumptions. If LGBTQ+ or other groups of employees were disproportionately represented in the non-promotion list, this is the starting point for further investigation.
A Machine Learning model can also be used to find similarities and differences between people who were promoted over a specific period. If there is over or under-representation of a particular group, that could be indicative of bias. Again, this is a starting point for further investigation, not a full-blow conclusion about bias.
It should be noted that although we use the example of LGBTQ+ identifying information, the same exercise could be run excluding gender or race information and then comparing the predicted promotions versus the actual. In this way, other potential areas of discrimination can be explored.
2. Use NLP to detect Individual harassment
LGBTQ+ employees are more likely to face sexual harassment at work.
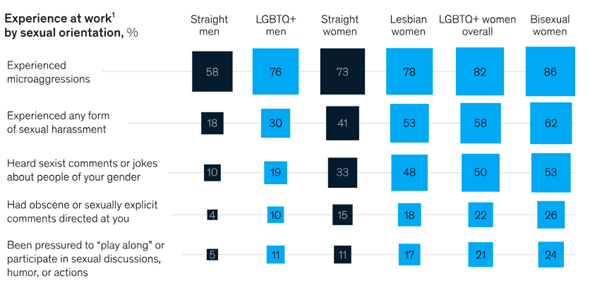
Source: McKinsey & Company
One way to flag aggressive online behaviors is by applying Natural Language Processing (NLP)-based models on the communication data which would flag toxic language or even more subtle micro-aggressions.
From a technical perspective, training a model to identify words or patterns of words is relatively easy to do. From an ethical perspective, applying AI to the content of individual employees’ communications is a lot more complex which is why the practice has not been broadly adopted.
It is likely that in the future, we will see limited (or carefully monitored) use of NLP to detect emerging patterns of discrimination. If done correctly, this could open the door to correcting behaviors and halting early signs of discrimination in its infancy.
3. Behavioral Predictive Analytics to identify disengagement and burnout
Another approach is to identify the talent risk of an entire organization, and then find ways to resolve the underlying cause at the individual level.
Let’s take the example of the company with 200 employees.
A Machine Learning algorithm extracts over 100 variables from both the HRIS and IT systems including tenure, age, email, and calendar usage. Without looking at the content (or applying NLP), algorithms are trained to look for normal behavior patterns and then to find signals of abnormal patterns. For instance, if a team works an average amount of hours and then there is a spike in hours worked, Behavioral People Analytics can be used to detect a burnout risk.

Source: Watercooler.ai
In the graph above, the team returns to normalized hours and the risk of burnout is reduced. In this case, there may have been an important deadline that required the additional hours and once the work was completed, people went back to their normal routines.
The type of analysis described here can be applied to multiple risk factors. For instance, the data can show that a person is becoming isolated from their team because the frequency of communication with team members is declining for one person, but not changing for the rest of the team. As with other examples, it should always be noted that human behavior is complex, and just because a model flags the potential for a specific behavior does not make it inevitable. Furthermore, there could be a good reason why someone is communicating less with their peers – they could be assigned a project that requires little input.
to If used carefully, Behavioral People Analytics can be used as a line of defense for LGBTQ+ employees. Let’s say that out of 200 employees, 15 people are identified as being at risk of isolation. With some irony, the journey to address issues of isolation of LGBTQ+ employees will take us a place where isolation risk should be analyzed for all employees, and then on a case-by-case basis, specific interventions be made to resolve the underlying issues.
Every organization will need to use this information differently and special care should be taken, especially if there is disengagement between an employee and their manager.
Summary and Conclusion
This article provides just a few examples of how AI can be used within the context of addressing LGBTQ+ discrimination.
For all the potential for AI, it is not a magic wand. LGBTQ+ employees need a safe space to work and opportunities to develop, both personally and professionally. AI will not create that tolerant workspace and it will not change attitudes or biases.
Human behavior is complex, and Behavioral People Analytics can be an enabler or tool. The use of AI alone is not the change that needs to happen. But what AI can do is significant. It can shine line in places that have been inaccessible: the day-to-day employee interactions, changes in compensation levels, and job promotions.
While we have a challenging journey ahead, it is crucial for all of us to join forces to protect the rights of the LGBTQ+ community and eliminate discrimination. It’s good for the companies we work for, but most importantly, it’s the right thing to do.
Related posts
More insights from Watercooler's team of experts.